Part 1: Digital twin for energy supply grid planning
With around 150 million tons of CO2 per year, transport is one of the largest emitters of climate-damaging greenhouse gases in Germany after the energy sector and industry. Therefore, it is no surprise that the expansion of electromobility is a key pillar of the transition to a climate-neutral transport system proclaimed by the federal and state governments. Cities in particular are focusing on expanding electromobility to achieve climate targets and reduce noise and particulate pollution – with some initial success. In 2022, the share of battery electric vehicles (BEV) and plug-in hybrid electric vehicles (PHEV) among new registrations has risen to around 31 percent[1]. With most manufacturers phasing out combustion technology over the next 10 to 15 years, experts expect the number of electric cars to rise to 11 million by 2030.[2]
Threat of grid overload due to massive increase in electromobility
This development poses major challenges for the various players in the energy industry, such as distribution grid operators. According to the grid operators, the number of electric vehicles currently being “refueled” via private wallboxes or public charging stations is already placing a considerable burden on the power grids.
In local distribution grids in particular, there is a risk of overloads due to temporary peaks in the event of a high number of simultaneous charging processes in the same grid segment. If the power demand exceeds the load limit of the grid segment, the segment is switched off as a precaution to avoid damage. This would result in temporary, local power failures.
Poor forecasting ability due to conventional tools
To counteract this, predictive network planning is needed that provides valid expansion scenarios to cope with the increasing capacity requirements in the course of the ongoing electrification of private transport. This is the challenge: While electricity grid planning has so far been able to accurately predict serious changes in grid load, the rapidly growing number of electric vehicles and increasing decentralized power generation are making this task massively more difficult. Three factors make planning more difficult:
- Private charging infrastructures:
For a reliable forecast of the expected load on the respective grid segments, the number of charging points available there in the future is crucial. A forecast of the public charging points does not in itself tell us much, since according to the BMVI (Network of Experts of the German Ministry of Transport and Digital Infrastructure) forecast, 60-85 % of the charging processes will take place on private property. Thus, no publicly available planning data is available for the crucial planning variable.
- Lack of location accuracy:
Especially in local distribution grids, the local distribution of the charging points is an important factor for assessing the load on subgrids. Although detailed models and solutions have existed for decades to simulate the local grid load for given expansion scenarios, there is no solution or data source to forecast and simulate the future expansion of electromobility on a local level (streets / apartment blocks). Especially for private households, central planning is not possible.
- Asynchronous expansion rates:
The regional and temporal distribution of e-vehicle acquisition also poses major challenges for electricity grid planning. Since the adoption rate of the technology is not evenly distributed throughout Germany, but depends heavily on factors such as age, gender, financial strength, residential environment, etc., future grid planning must take these environmental factors into account. This heterogeneous distribution is also reinforced by a dynamic development in the registrations of company e-cars.
As a result, there is a lack of valid expansion scenarios to calculate detailed planning for future grid design. In other words, the challenges of e-mobility cannot be solved by local distribution grid operators with existing tools.
New approaches through digital twins
Against this background, the digital twin offers new solutions for forecasting future energy demand at the level of individual buildings or street sections. In a pilot project, m3 management consulting has developed a digital twin together with various partners, which forecasts the additional grid load due to the expansion of private wallboxes up to the year 2040 in the grid area with address accuracy.
For this purpose, a digital platform was set up in which, in addition to public charging infrastructure elements, private wallboxes and – if available – photovoltaic systems are also mapped. The information obtained in this way is enriched with technical and socioeconomic data, such as income structure, age structure, and building information. With the help of a multi-stage AI process, the deployment of new wallboxes and the associated additional grid load in the coming years is forecast with address accuracy

The solution thus enables predictive network development along regional requirements and early indications of cost-optimized strategic planning with a high degree of flexibility. At the same time, the system can be extended to include other use cases by integrating additional data sources, such as the forecasting of heat pumps or the development of decentralized battery storage systems. In particular, the addition of mobile battery storage will enable the simulation of vehicle-to-grid operations to perform in-depth analyses.
Further use cases and necessary framework conditions
The example clearly illustrates the potential that the digital twin offers in terms of transparency and forecasting capability for existing and future distribution grids in the context of the transition to a climate-neutral transport system. In addition to grid expansion, this relates to other application examples, such as the support of grid-serving charging through the intelligent exploitation of flexibilities in electricity demand or automated grid connection tests for private or public charging points.
In addition to a satisfactory data basis and data quality, a prerequisite for successful implementation is comprehensive change management and an adjustment of processes and structures in the organization of grid operators toward more cross-functional cooperation models.
Sources:
[1] Agora Verkehrswende (2023): https://www.agora-verkehrswende.de/veroeffentlichungen/marktentwicklung-von-e-autos/
[2] Center of Automotive Management (2022) https://auto-institut.de/wp-content/uploads/2022/12/STUDIE_Mobilitaet_der_Zukunft_2022_BNP-Paribas-Cardif_CAM.pdf
About "Smart Energy City"
On the path to sustainable and future-oriented urban development, municipalities will face enormous energy challenges in the coming years. Whether it is the sustainable expansion of district heating and district cooling networks to achieve climate targets in the building sector or electricity grid planning to cope with increasing grid loads due to the requirements of e-mobility – many of the future challenges cannot be solved by local distribution grid operators using the existing tools.
In our two-part article “Smart Energy City”, you’ll learn how digital twins can provide new solutions in this context and thus become a key pillar for the smart and climate-neutral city of tomorrow:
Find out more.
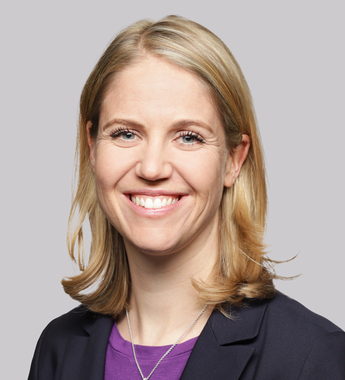
Katrin Aytepe
Managing Director
We are looking forward to contacting you!
Further topics
-
Smart Energy City -
Part 2
In our two-part article “Smart Energy City”, you’ll learn how digital twins can provide new solutions in this context and thus become a key pillar for the smart and climate-neutral city of tomorrow.
- 1
- 1
- 1